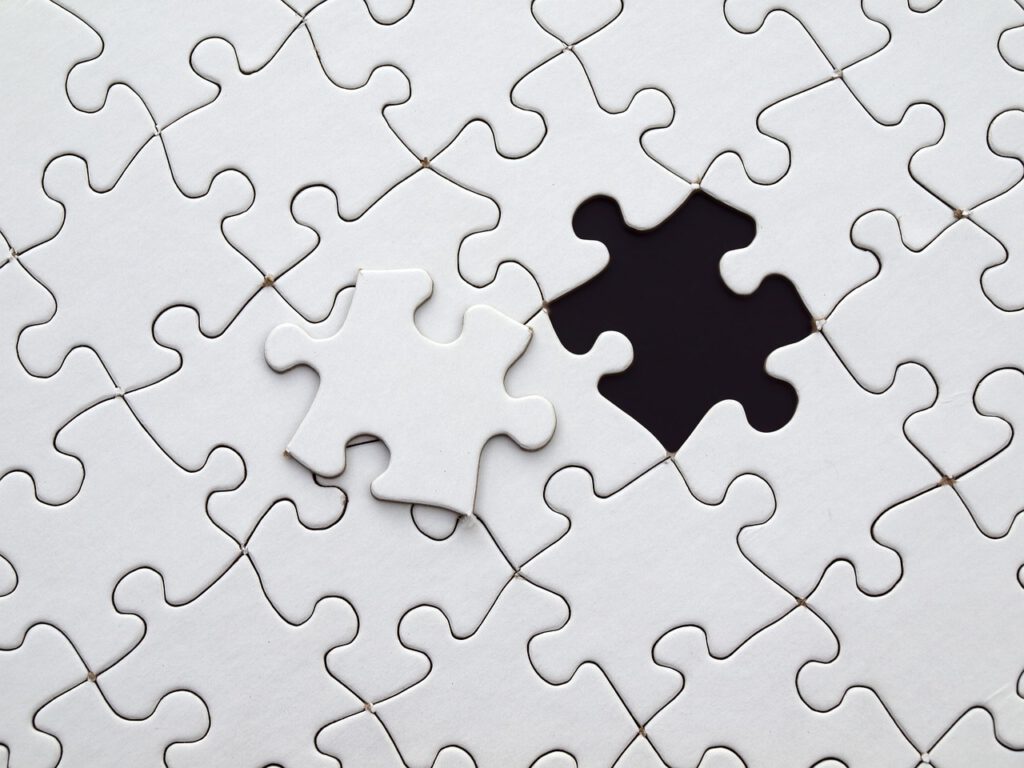
How to build better AML/ CTF systems?
As financial crimes evolve, so must the systems designed to combat them. At anti-money-laundering.eu, we focus on the innovative strategies and approaches essential for developing more effective Anti-Money Laundering (AML) and Counter-Terrorist Financing (CTF) systems. Here, we outline the key elements that contribute to building stronger, more resilient AML/CTF frameworks.
1. Enhancing Data Availability and Quality: The foundation of any robust AML/CTF system lies in the quality and accessibility of data. Ensuring access to comprehensive, accurate, and up-to-date financial data is critical. This involves establishing partnerships with data providers, investing in data aggregation tools, and implementing stringent data quality control measures.
2. Staying Ahead of Criminal Methodologies: To effectively counter financial crimes, AML/CTF systems must be dynamic and adaptive, constantly updating their knowledge base with the latest criminal methodologies and typologies. This requires ongoing research, collaboration with law enforcement agencies, and integration of real-world case studies into system algorithms.
3. Leveraging Big Data Analytics: Harnessing the power of Big Data analytics is vital for processing and analyzing vast volumes of financial transactions. Investing in advanced analytical tools and technologies, such as machine learning and artificial intelligence, can significantly enhance the system’s ability to detect and flag suspicious activities in real time.
4. Fine-Tuning Detection Algorithms: Developing sophisticated algorithms capable of identifying both known and emerging laundering patterns is essential. Regular calibration and testing of these algorithms can help reduce false positives and negatives, improving the overall efficiency and reliability of the system.
5. Incorporating Detailed Human Feedback: Human expertise and feedback are invaluable in refining AML/CTF systems. Establishing clear channels for feedback, and ensuring that this feedback is specific, actionable, and integrated into system updates, can greatly enhance the system’s accuracy and responsiveness.
6. Implementing Advanced Learning Mechanisms: Incorporating both supervised and unsupervised learning mechanisms allows AML/CTF systems to continuously evolve and adapt. By learning from historical data and human interactions, these systems can become more adept at identifying complex and subtle patterns of financial crimes.
7. Regularly Conducting System Re-Runs: Periodically re-running past data through updated algorithms is crucial for validating improvements and identifying areas that require further refinement. This practice ensures that the system remains effective against the ever-changing landscape of financial crimes.
Building better AML/CTF systems is a multifaceted endeavor that demands attention to data quality, technological advancement, and continuous learning and adaptation. At anti-money-laundering.eu, we are dedicated to providing insights, resources, and innovative approaches to strengthen AML/CTF efforts worldwide. Join us in our mission to develop systems that are not only effective but also resilient in the face of evolving financial threats.