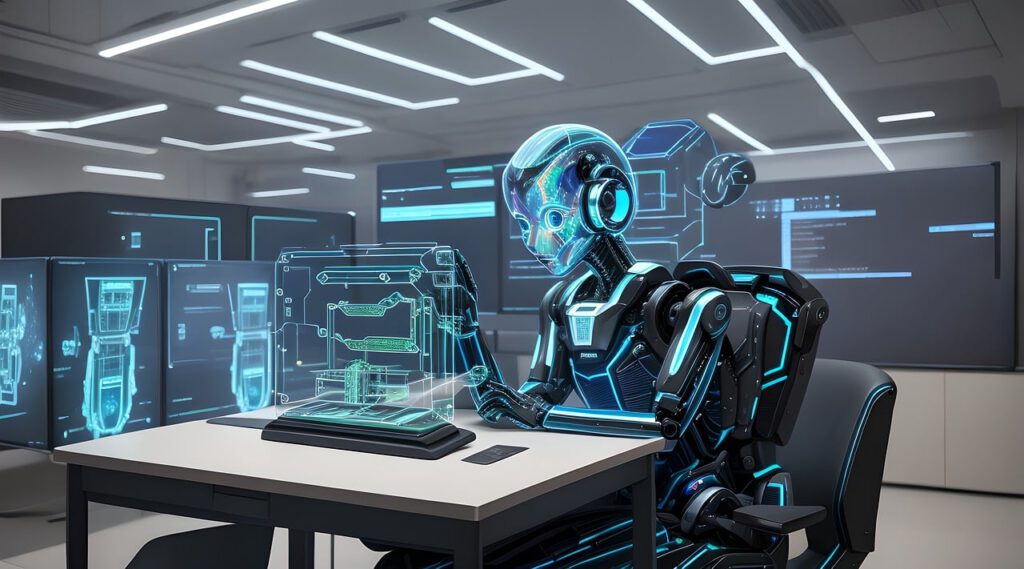
Advanced Analytics
Advanced Analytics refers to the autonomous or semi-autonomous examination of data or content, using sophisticated techniques and digital tools, typically beyond those of traditional business intelligence, to discover deeper insights, make predictions, or generate recommendations.
Advanced Analytic Techniques (Advanced Analytics) include those such as data/text mining, machine learning, pattern matching, forecasting, visualisation, semantic analysis, sentiment analysis, network and cluster analysis, multivariate statistics, graph analysis, simulation, complex event processing, neural networks.
Advanced Analytics typically rely on the use of big data.
Advanced Analytics is revolutionizing the field of Anti-Money Laundering and Countering the Financing of Terrorism (AML/CFT). By harnessing a spectrum of sophisticated techniques, it empowers financial institutions to delve deeper into data, uncover hidden insights, and stay ahead of financial criminals.
Let’s explore the key components of Advanced Analytics and their roles in enhancing AML/CFT strategies.
- Data/Text Mining: A technique that processes huge volumes of data to extract meaningful information, crucial for spotting trends and patterns in financial transactions.
- Pattern Matching: This technique identifies and flags transactions that exhibit characteristics of known illicit activities, aiding in prompt detection of potential risks.
- Forecasting: Uses historical data to predict future trends, enabling financial institutions to anticipate and prepare for potential money laundering activities.
- Visualization: Transforms complex data sets into intuitive visual formats, making it easier to spot unusual patterns indicative of criminal activity.
- Semantic Analysis: Focuses on understanding the meaning and context of words within financial communications, aiding in the detection of suspicious narratives.
- Sentiment Analysis: Assesses the tone and sentiment behind textual data, adding an extra layer of scrutiny to financial communications.
- Network and Cluster Analysis: Analyzes how entities are interconnected, revealing clusters or networks indicative of illicit financial rings.
- Multivariate Statistics: Examines multiple variables simultaneously to understand complex relationships in financial data.
- Graph Analysis: Involves visualization and analysis of data in graph form, essential for mapping financial networks and flows.
- Simulation: Models real-world financial scenarios to study the behavior and dynamics of systems, predicting potential risks.
- Complex Event Processing: Enables real-time monitoring and analysis of financial transactions to identify suspicious activities instantly.
- Neural Networks: Leverage AI for pattern recognition in vast datasets, excelling in detecting sophisticated money laundering techniques.
Advanced Analytics in AML/CFT is not just a tool; it’s a game-changer. It represents a shift from traditional reactive methods to proactive, data-driven strategies. By integrating these techniques, financial institutions can enhance their capabilities to detect, analyze, and prevent financial crimes more effectively than ever before.
Sources: