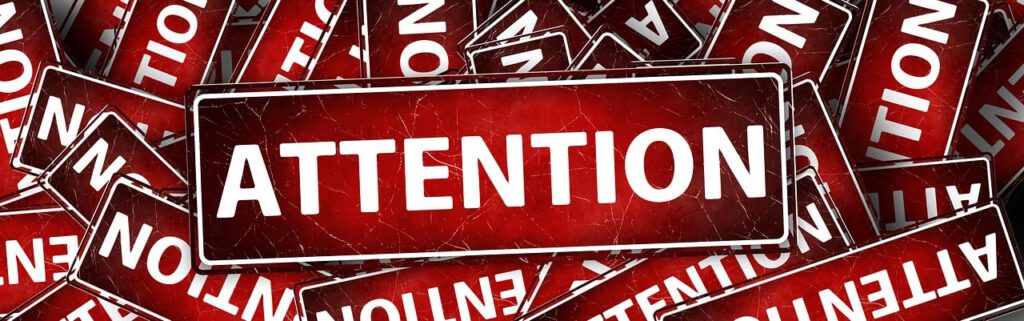
The hidden danger of Machine Learning in AML/CTF Risk Models
While Machine Learning (ML) holds significant promise in enhancing anti-money laundering (AML) and counter-terrorist financing (CTF) efforts, it’s crucial to be aware of the potential dangers that come with its implementation in risk models.
Over-Reliance on Automation: One of the primary dangers of ML in AML/CTF risk models is over-reliance on automation. While ML algorithms can efficiently process data, there’s a risk of neglecting the human element. Human expertise and intuition remain invaluable in detecting nuanced financial crimes that algorithms may overlook.
Data Bias and Discrimination: ML models are only as good as the data they are trained on. If historical data used for training contains biases, the ML algorithms may perpetuate these biases, leading to discrimination and inaccurate risk assessments. It’s essential to constantly monitor and address bias in the data and algorithms.
Complexity and Lack of Transparency: ML algorithms can be complex and challenging to interpret. This lack of transparency can make it difficult for AML/CTF investigators and regulatory authorities to understand and explain the reasoning behind ML-generated alerts. Ensuring transparency in ML models is crucial for compliance and accountability.
Adversarial Attacks: Criminals are becoming increasingly sophisticated in their techniques. ML models are vulnerable to adversarial attacks, where malicious actors manipulate inputs to evade detection. Financial institutions must invest in robust security measures to protect against such attacks.
Regulatory Challenges: The regulatory landscape for ML in AML/CTF is still evolving. Financial institutions must navigate the complexities of compliance, ensuring that their ML-driven risk models meet regulatory requirements. Staying informed about changing regulations is essential to avoid penalties and reputational damage.
Balancing Efficiency and Accuracy: While ML can improve efficiency in AML/CTF efforts, there’s a delicate balance between efficiency and accuracy. Over-optimization for efficiency can lead to an increased risk of false negatives, missing genuine threats. Striking the right balance is crucial.
In summary, the use of Machine Learning in AML/CTF risk models brings both promise and peril. Financial institutions must tread carefully, combining the power of ML with human expertise, addressing data bias, ensuring transparency, and safeguarding against adversarial attacks. By understanding and mitigating these dangers, organizations can harness the full potential of ML while maintaining effective AML/CTF efforts and compliance with regulatory requirements.