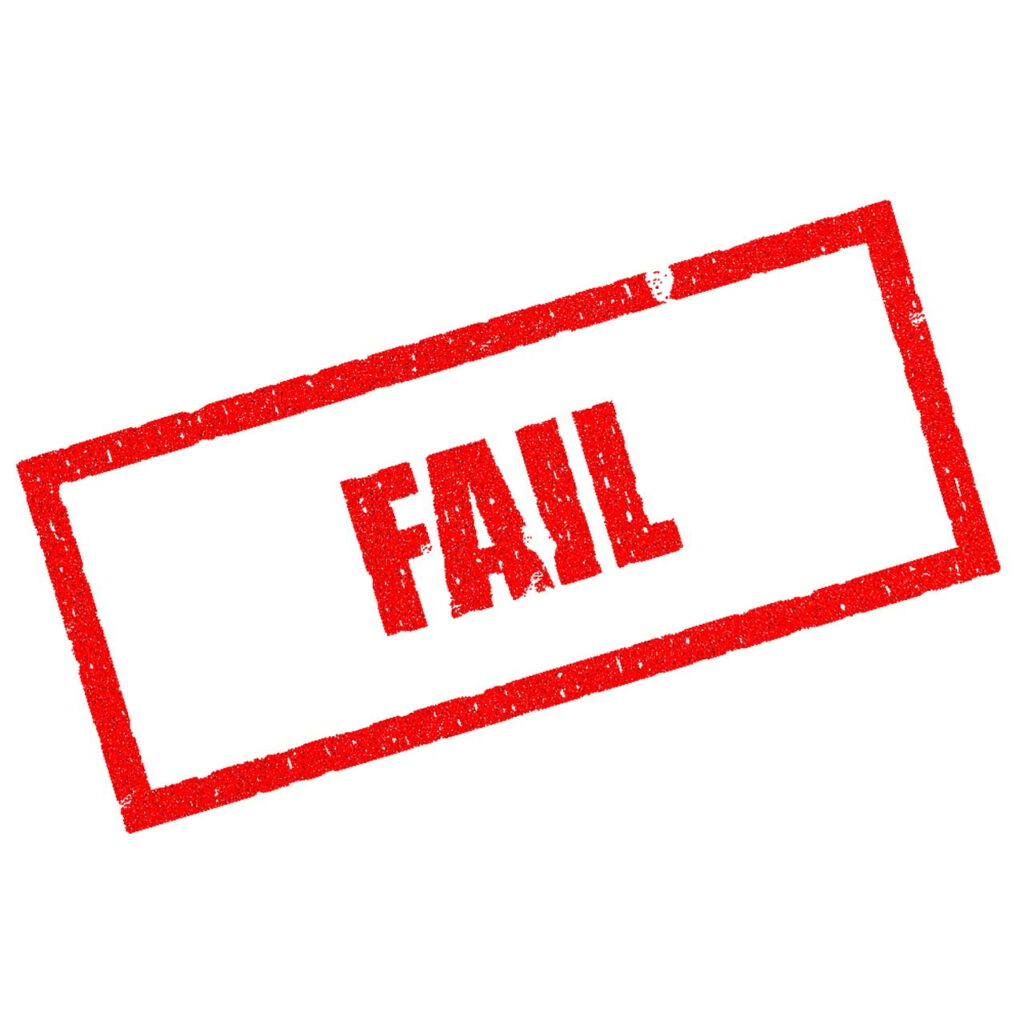
Why most AML/CTF systems fail?
In today’s rapidly evolving financial landscape, Anti-Money Laundering (AML) and Counter-Terrorist Financing (CTF) systems play a crucial role in safeguarding the integrity of financial institutions. However, despite their significance, most AML/CTF systems face challenges that hinder their effectiveness. At anti-money-laundering.eu, we delve deep into the reasons why most AML/CTF systems fail to meet the expectations.
1. The Challenge of Data Availability and Quality: AML/CTF systems are heavily reliant on the availability and quality of data. Often, these systems grapple with accessing comprehensive and up-to-date financial data, limiting their ability to accurately track and analyze transactions. Additionally, issues like data inaccuracy, inconsistencies, and outdated information can lead to erroneous conclusions and hinder effective monitoring.
2. Keeping Up with Criminal Methodologies: The landscape of money laundering and terrorist financing is continuously evolving, with criminals consistently devising new methods to evade detection. Many AML/CTF systems struggle to stay updated with these changing typologies, rendering them ineffective against novel or sophisticated laundering techniques.
3. Big Data Processing Capabilities: The ability to process and analyze large volumes of data in real time is paramount for AML/CTF systems. However, handling the vast amount of financial transactions, often referred to as ‚Big Data‘, poses significant technical challenges, impacting the systems‘ ability to detect suspicious activities efficiently.
4. Detection and Analysis Limitations: Despite being equipped with algorithms to identify known laundering patterns, most AML/CTF systems face difficulties in discerning subtle or complex suspicious activities. This limitation often leads to a failure in detecting nuanced typologies of money laundering and terrorist financing within the available data.
5. Calibration and Logic of Search Algorithms: The calibration of search algorithms, including aspects like fuzzy logic, greatly influences the effectiveness of AML/CTF systems. An imbalance in this calibration can result in a high rate of false positives or negatives, either overwhelming the system with irrelevant alerts or missing genuine suspicious activities.
6. The Need for Specific Human Feedback: Human input plays a critical role in refining AML/CTF systems. However, the lack of specific, detailed feedback on the system-generated alerts often leads to missed opportunities for system improvement and increased accuracy.
7. Inadequate Learning Mechanisms: Most AML/CTF systems exhibit a lack of advanced learning mechanisms, such as supervised and unsupervised learning from human-handled alerts. This drawback limits their ability to evolve and adapt to new patterns and typologies of financial crimes.
8. The Absence of Re-Runs Post-Feedback: Re-running past data through updated algorithms is a crucial step in validating and enhancing the system’s performance. Unfortunately, this is often neglected due to resource constraints, leaving potential improvements untested.
The failure of most AML/CTF systems can be attributed to a combination of data-related challenges, technological limitations, and an inability to adapt to the dynamic nature of financial crimes. At anti-money-laundering.eu, we are committed to exploring and addressing these challenges, offering insights and solutions to enhance the effectiveness of AML/CTF efforts. Join us in our quest to strengthen financial security and combat financial crimes more effectively.